-
Aviation Advisory
Our dedicated Aviation Advisory team bring best-in-class expertise across modelling, lease management, financial accounting and transaction execution as well as technical services completed by certified engineers.
-
Consulting
Our Consulting team guarantees quick turnarounds, lower partner-to-staff ratio than most and superior results delivered on a range of services.
-
Business Risk Services
Our Business Risk Services team deliver practical and pragmatic solutions that support clients in growing and protecting the inherent value of their businesses.
-
Deal Advisory
Our experienced Deal Advisory team has provided a range of transaction, valuation, deal advisory and restructuring services to clients for the past two decades.
-
Forensic Accounting
Our Forensic and Investigation Services team have targeted solutions to solve difficult challenges - making the difference between finding the truth or being left in the dark.
-
Financial Accounting and Advisory
Our FAAS team designs and implements creative solutions for organisations expanding into new markets or undertaking functional financial transformations.
-
Restructuring
Grant Thornton is Ireland’s leading provider of insolvency and corporate recovery solutions.
-
Risk Advisory
Our Risk Advisory team delivers innovative solutions and strategic insights for the Financial Services sector, addressing disruptive forces, regulatory changes, and emerging trends to enhance risk management and foster competitive advantage.
-
Sustainability Advisory
Our Sustainability Advisory team works with clients to accelerate their sustainability journey through innovative and pragmatic solutions.
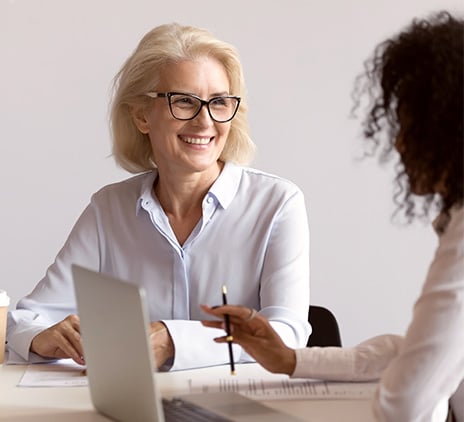
-
Asset management Asset management of the futureIn today’s global asset management landscape, there is an almost constant onslaught of change and complexity. To combat such complex change, asset managers need a consolidated approach. Read our publication and find out more about what you can achieve by choosing to work with us.
-
Internal Audit Maintaining Compliance with New EU Pension Directive IORP IIOn 28 April 2021, the Irish Government transposed IORP II (Institution for Occupational Retirement Provision), an EU directive on the activities and supervision of pension schemes, into law.
-
Risk, Compliance and Professional Standards FRED 82 – Periodic Updates to FRS 100 – 105The concept of a new suite of standards for the UK and Ireland, aligning with international financial reporting standards, was first conceived in 2002
-
Audit and Assurance Auditor transition: how to achieve a smooth changeoverAppointing new auditors may seem like a daunting task that will be disruptive to your business and a drain on the finance function. Nevertheless, there are a multitude of reasons to consider a change, including simply seeking a ‘fresh look’ at the business.
-
Corporate Tax
Our Corporate Tax team is made up of more than 40 highly experienced senior partners and directors who work directly with a wide range of domestic and international clients; covering Corporation Tax, Company Secretarial, Employer Solutions, Global Mobility and Tax Incentives.
-
Financial Services Tax
The Grant Thornton team is made up of experts who are fully up to date in terms of changing and evolving tax legislation. This is combined with industry expertise and an in-depth knowledge of the evolving financial services regulatory landscape.
-
International Tax
We develop close relationships with clients in order to gain a deep understanding of their businesses to ensure they make the right operational decisions. The wrong decision on how a company sells into a new market or establishes a new subsidiary can have major tax implications.
-
Private Client
Grant Thornton’s Private Client Services team can advise you on all areas of financial, pension, investment, succession and inheritance planning. We understand that each individual’s circumstances are different to the next and we tailor our services to suit your specific needs.
-
VAT
Grant Thornton’s team of indirect tax specialists helps a range of clients across a variety of sectors including pharmaceuticals, financial services, construction and property and food to navigate these complexities.
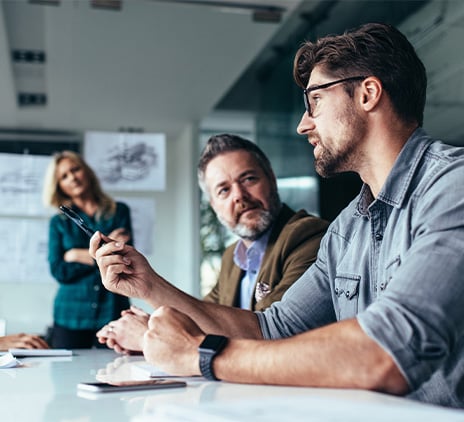
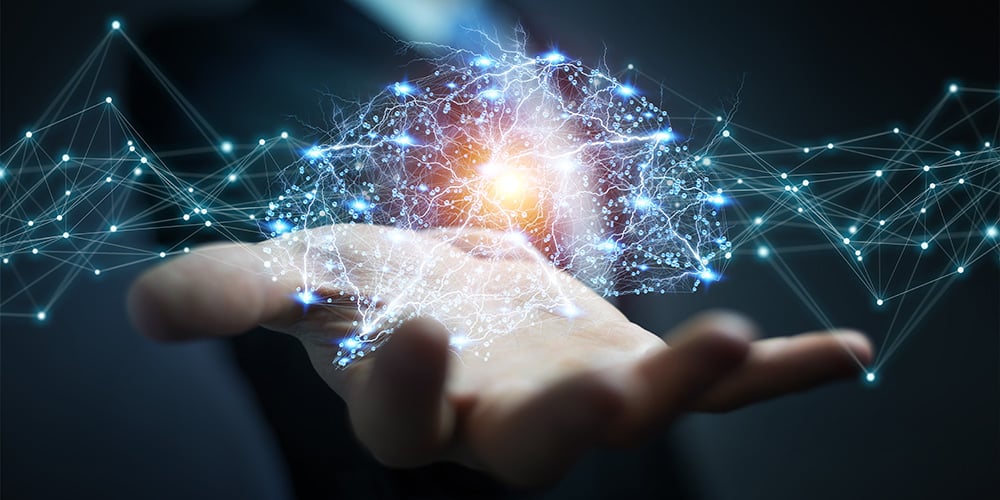
Background
Modelling techniques for bank risk management have always been an important element, with greater emphasis during the last two decades. Well established risk quantification methods are used by banking institutions within their capital calculation, provisioning, forecasting and stress testing, pricing and decision making.
The significant improvement in data processing and computational capabilities has resulted in an increasing industry trend to use more advanced techniques in risk identification and quantification. The trend has been strongest in the area of decision making (non-regulatory) models.
However, recent publications on regulatory models from the EBA (European Banking Authority) and PRA (Prudential Regulation Authority) are developing advanced method use cases. An increased focus on Artificial Intelligence (AI) and machine learning (ML) methods highlights the need for bank risk management to understand the capabilities of advanced modelling techniques.
Within Credit Risk, a role for ML models is becoming more and more relevant across several areas. Examples include:
- Deciding how to categorise or rank order loans or borrowers;
- Identifying the cohort of loans that are most vulnerable;
- Designing efficient credit sanctioning and review processes and
- Identifying emerging risks in a dynamic way.
Having the ability to process data efficiently and help institutions to make better informed decisions in a timely manner represents a few benefits of advanced modelling techniques.
Executive Summary
Our focus in this paper is to develop decision making models using a range of advanced machine learning techniques. We explore three different methodologies to measure the discriminatory power between good and bad borrowers using a credit card portfolio dataset. The main hypothesis is that advanced modelling techniques lead to more efficient estimates and higher discriminatory power.
The first section reviews the applicable regulatory information. Recent regulatory publications and initiatives acknowledge a need to address advanced modelling techniques use cases in bank risk management. A focus on the regulatory capital models shows a relatively conservative regulatory position. The second section provides the definitions on decision making and machine learning principles.
In the third section, three models with varying complexity levels are applied to the credit card dataset. An assessment is performed on the ability to discriminate borrowers repayment status between good (performing) and bad (nonperforming). Model output confirms the hypothesis that applying more advanced ML techniques significantly improves model discriminatory power, leading to more efficient rank ordering. A final section provides a benefit analysis (pros and cons) of using ML techniques in this area.
Read the full publication as we explore three different methodologies to measure the discriminatory power between good and bad borrowers using a credit card portfolio dataset.