Sign up for expert insights, industry trends, and key updates—delivered straight to you.
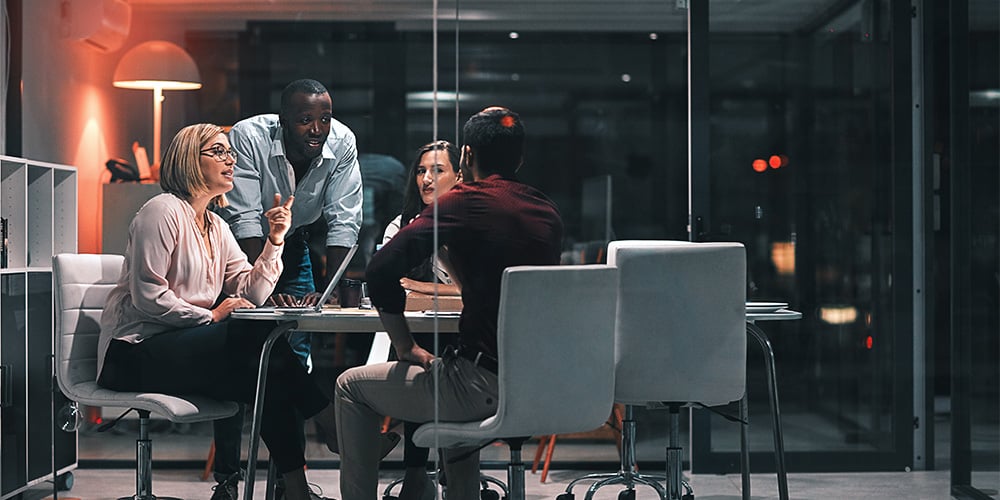
As an industry built on data, where investor demands are growing and regulations are evolving, fund administration is ideally positioned to benefit from artificial intelligence. By automating manual processes, streamlining documentation, flagging risks and answering queries, AI can bring unprecedented efficiency and effectiveness to fund administrators’ roles. But before they can embrace AI, fund administrators must undertake a strategic digital transformation.
Digital transformation integrates technology into all areas of a business, revolutionising how organisations operate. It means adopting new tools and platforms, automating processes and making data-led decisions. This improves customer experience and drives innovation organisation-wide. The digital transformation journey must be embraced at all levels of the organisation starting with executive leadership. This ensures alignment to strategic goals and can serve as a key driver for optimal results.
A role that’s ripe for change
Traditionally, as fund administrators independently maintain the books and records for funds, their systems remain siloed within the funds’ overall ecosystem. But recent developments have expanded and complicated their roles.
Consolidation within the industry
The fund administration space has gone through a period of consolidation over the past few years, after a wave of M&A activity. Integrating acquired fund administration businesses, their systems and operating procedures has been challenging given the pace at which M&A has taken place. There is a need to decide on best-in-class tech stacks and operating procedures and execute on projects to integrate them.
Evolving regulatory reporting requirements
AIFMs, management companies and investment managers increasingly outsource regulatory reporting obligations to fund administrators. Administrators must be agile to keep up with evolving regulations. Reliance on externally licensed systems, with upgrades at the mercy of vendor’s priorities, means many reports are developed offline—a time-consuming and error-prone process.
An increase in investor quantum and demands
Investor demand for alternative assets has grown rapidly in the last decade, with no sign of slowing down. According to a 2024 Bain & Company analysis, private market assets are expected to reach USD60-65 trillion by 2032, growing twice as fast as public assets.
Institutional investors previously dominated this space, meaning the number of investors per fund was typically low. An increasing appetite among retail investors for alternative portfolio assets has increased the quantum of investors per fund. This creates challenges for fund administrators, with more investors to onboard and the need to clear KYC/AML requirements and fulfil periodic reporting.
These tasks historically required manual input, scanning documents and extensive communication between investors and administrators’ investor relations and AML teams. But the added volume and workload have not translated into increased fees.
Contraction in reporting cycles
Investment managers face pressure from investors to report more often and more transparently. This means producing more frequent net asset value (NAV) calculations and investor reporting suites, creating pressure to streamline the NAV process. Additional reporting requirements are often addressed manually, requiring bespoke requests to external software vendors or internal development teams, which can lead to deployment delays.
Hybrid fund structures offering flexibility
The industry has seen a rise in fund structures offering hybrid liquidity options for investors. Many funds offer a blend of capital commitments and further ongoing open-ended opportunities to invest. Capital, therefore, can’t simply be divided into open- or closed-ended funds anymore. Additionally, many funds now invest in both liquid and illiquid investments.
Selecting fund accounting systems in this context is about compromise. Systems must cater to most of the fund’s parameters—investor capital structure and related fee calculations versus types of portfolio holdings. The rest must be handled by workarounds in and outside systems.
Increased data volumes
The rise in algorithmic or automated trading in quant funds and cryptocurrency has massively increased trade volumes. These funds use AI agents to trade on market inefficiencies, often trading the same security multiple times per day. This volume puts strain on administrators’ systems. Robust tools are needed for data transformation and ingestion but require extra system resources.
Why digital transformation is the answer
Digital transformation can make information work more efficiently across the value chain, driving smarter decisions and underpinning a robust data strategy. It’s also an essential first step toward harnessing the power of AI.
Collaboration across the value chain
Fund administrators handle vast amounts of data that can offer valuable insights to asset managers, helping inform decisions on investment and distribution.
Broader digital transformation that includes the entire value chain, from front- to back-office systems, can optimise and integrate all aspects of an organisation. This improves efficiency and effectiveness across the board.
Fund administrators must view themselves as integral partners in the value chain, for all relevant departments. This allows them to gain a more comprehensive perspective, ensuring more fluid strategy and implementation of digital transformation.
Fund administrators can also alleviate the reporting burden on investment managers by automating day-to-day client requirements. The key is to minimise manual data intervention and focus on value-add functions. Moving toward exception-based reviews can improve data management, efficiency and accuracy.
Becoming data-driven
Being data-driven means integrating data and analytics into every aspect of the business. This involves fostering a culture that understands and effectively manages data. And establishing data governance frameworks is crucial to maintain data accuracy, consistency and security.
Data driven also entails obtaining access to data and breaking technical silos that restrict data access, in addition to establishing a common data dictionary and language to extract data insights.
Fund administrators should embed data-driven insights into decision-making processes. This ensures they become more agile and responsive to market changes, helping to increase efficiency, reduce costs and open revenue streams. Data can unlock new opportunities, optimise processes and enhance client experiences, creating a competitive edge.
Defining a data strategy
A well-defined data strategy is crucial to unlocking the full potential of data. This includes understanding the value of data, managing it effectively and using it to drive business goals. A data strategy should include:
- Data governance
- Policies, standards and procedures to ensure data quality, security and compliance
- This involves not only adhering to regulatory frameworks such as GDPR, but also setting standards for data accuracy, consistency, and reliability.
- Data management
- Systems and processes to collect, store and analyse data efficiently
- This includes both big data and small data, as small data can provide detailed insights that are often overlooked.
- Integration into BAU
- Data and analytics integrated into operations for informed decision-making resulting in more accurate reporting produced by administrators.
Unlocking the potential of AI
Digital transformation changes how an organisation operates and delivers customer value. What’s more, it unlocks AI capabilities enabling fund administrators to automate tasks, gain deeper insights from data and increase reporting accuracy.
According to Eurostat, enterprises with 250+ employees—including most global fund administrators—are increasingly adopting AI. Over 21% of them use AI for text mining and language analysis, a 9.5% increase from 2023. This is followed by the adoption of machine learning, with almost 21% adoption rate and 6% increase year on year.
AI technology | % 2024 | % 2023 | Change |
---|---|---|---|
Enterprises using AI technologies performing analysis of written language (text mining)
|
21.44
|
11.90
|
9.54
|
Enterprises using AI technologies converting spoken language into machine-readable format (speech recognition)
|
16.70
|
9.11
|
7.59
|
Enterprises using AI technologies generating written or spoken language (natural language generation)
|
16.64
|
7.73
|
8.91
|
Enterprises using AI technologies identifying objects or persons based on images (image recognition, image processing)
|
13.40
|
10.48
|
2.92
|
Enterprises using machine learning (e.g. deep learning) for data analysis
|
20.58
|
14.62
|
5.96
|
Enterprises using AI technologies automating different workflows or assisting in decision making (AI based software robotic process automation)
|
20.40
|
16.42
|
3.98
|
Enterprises using AI technologies enabling physical movement of machines via autonomous decisions based on observation of surroundings (autonomous robots, self-driving vehicles, autonomous drones)
|
7.19
|
7.01
|
0.18
|
AI improves data accuracy through automated data cleansing, detecting anomalies in real-time and providing personalised recommendations and reporting to investment managers. Additionally, AI can optimise investment strategies, enhancing risk management and streamlining compliance processes.
AI use cases in fund administration
Many organisations recognise the potential of large language models (LLMs) for assisting and supplementing daily tasks. ChatGPT is an example of an LLM which has replaced the function of a search engine and can help with writing or idea generation in our everyday lives. Some companies use closed LLMs like Microsoft’s Copilot or are developing in-house equivalents. They are starting to connect these models to their own data sources and knowledge libraries to drive experimentation amongst their workforces.
Use cases of AI with special interest to fund administrators include:
AI agents can scrape and summarize data in investor application forms and KYC documents. This frees time for investor services/AML teams to analyse outputs in the onboarding process. AI-enabled AI agents can also monitor transactions and flag suspicious activity. AI can be further utilized for risk management in identifying illicit/fraud activity in transaction data and enable risk modelling scenarios for fund activities.
Founding documents like PPMs, prospectuses and LPAs are lengthy documents. Fund administrators can extract all relevant information to set up funds in transfer agency systems—but this is time-consuming, resource-heavy and prone to human error. AI agents can analyse documents, distil and extract relevant fund information which can then be reviewed by administrators before entering into administration systems.
Where a fund invests in underlying funds or companies, it’s often necessary to carry additional data in the administration system. AI agents can extract and summarize relevant information.
Fund administrators consume vast quantities of data into different applications and systems, making it difficult to consolidate information. Administrators therefore use multiple fund accounting systems, chosen based on their strengths and weaknesses in processing or reporting on the specific structures and parameters of different funds. AI automates data aggregation, eliminating reliance on the default tool—spreadsheets.
AI, which can be embedded into those spreadsheets, has the capability to streamline and improve the accuracy of complex calculations such as performance fees, catch-up, waterfall distributions or equalization. AI algorithms can process vast amounts of data swiftly, ensuring that all calculations adhere to the fund terms outlined in fund documents like PPMs for Private Equity funds and can also consider other investor specific data.
AI can also automate the client billing process by interrogating databases for fee accruals as recorded in the NAVs of their fund accounting systems. This can improve turnaround in fee collection and remove the need for manual capture.
Traditional NAV calculations for mutual and hedge funds often rely on T+1 or even T+2 processing due to manual reconciliations, pricing adjustments, and data validation. AI-driven systems can aggregate and validate trade pricing and position data in real time enabling faster NAV finalisation.
The holy grail of fund accounting has always been to achieve real-time, fully automated and error-free NAV calculations and now there is a tool to help make this a reality.
Enriching the reporting suite available to both asset managers and investors has become a point of differentiation amongst fund administrators. AI insights can be utilised to personalise reporting and performance dashboards according to individual preferences, retrieving more meaningful reporting and analysis to optimise client experience.
Fund administrators carry a large amount of personal data. This heightens the obligation to protect against cyberattacks. AI can enhance cybersecurity by monitoring access logs and flagging suspicious login attempts. This mitigates the risk of punitive GDPR-related fines and reputational damage.
Financial services institutions use their own LLMs to query databases and address client queries. Centralising this knowledge decreases queries between departments and reduces the time needed to address them.
Many use chat bots to address initial client queries around the clock. They are becoming increasingly sophisticated, and they improve with each interaction.
AI implementation challenges
There are several hurdles to overcome for implementation to be successful, but fund administrators can address them with foresight and thoughtful planning. Key strategies include:
Anticipating cultural issues
Adopting AI requires a shift in perspective; leadership must manage changes appropriately to ensure buy-in. Technological disruption often creates skepticism and fears around AI replacing roles as opposed to assisting or streamlining workflows.
Open AI’s first LLM GPT-1, released in 2018, had around 100 million parameters. By 2023, GPT-4 had over a trillion parameters – around a 10,000-fold increase in model complexity. Companies using AI must be agile and employees must understand the technology to make the most of its opportunities.
Ensuring data quality
‘Garbage in, garbage out’ applies to AI-driven processes where structured data is required. Successful implementation must be preceded by a clean-up of source data to improve results. This is particularly true for historical data, which may have evolved over time.
Futureproofing risk frameworks and controls
Robust governance frameworks are needed to ensure risks are managed. Models must be properly documented, defined and explainable. Privacy regulations must be followed, and sensitive information must be protected within the ecosystem. A readiness assessment in line with operational, regulatory and risk controls must be conducted before rolling out AI-assisted technology.
Regulations are playing catch-up with AI development and may lag behind future advances. The EU AI Act, published in July 2024, considers personal data processing as high risk and to be closely scrutinised—something for fund administrators who process and maintain sensitive investor data, to keep top-of-mind.
Conclusion: It’s time to transform
While AI adoption in fund administration is still in its infancy, it has already created opportunities to supplement and enhance processes across workstreams.
Fund administrators can harness AI to innovate, improve efficiency and create growth opportunities. The sooner they do so, the more of an edge they’ll have over competitors. But the first step toward the AI future is a well-planned digital transformation—one that recognises the power of data to drive your business forward.